Leveraging Data Annotation in Machine Learning for Business Success
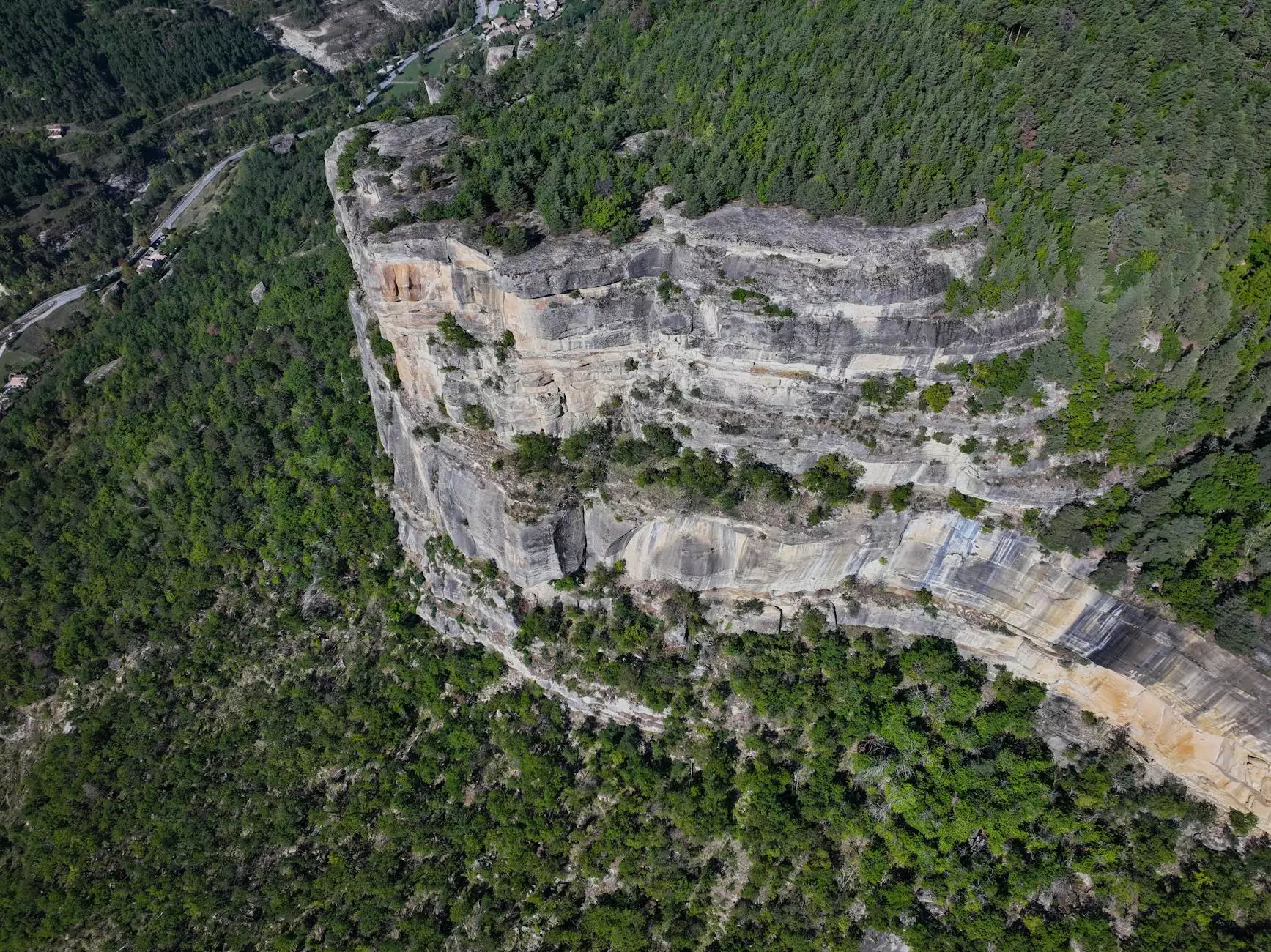
The modern business landscape is constantly evolving, driven by the advancements in technology and the growing importance of data. Among the most pivotal advancements is the rise of machine learning, a branch of artificial intelligence that enables computers to learn from data and make decisions based on it. A crucial component of machine learning is data annotation, which is essential for training algorithms effectively. This article will delve into the significance of data annotation machine learning and how businesses can harness its power to thrive in an increasingly digital world.
The Importance of Data Annotation in Machine Learning
Data annotation involves labeling and tagging data to provide context and meaning, allowing machine learning models to understand the information correctly. Without accurately annotated data, machine learning models can misinterpret the information, leading to erroneous outcomes. Here are several reasons why data annotation is critical for businesses:
- Improved Accuracy: Properly annotated data significantly enhances the accuracy of machine learning models, enabling them to make more reliable predictions.
- Efficient Training: Annotated datasets allow models to train efficiently, reducing the time required to achieve desired outcomes.
- Better User Experiences: Businesses can leverage machine learning to personalize customer interactions by utilizing well-annotated data, leading to improved user satisfaction.
- Cost-Effectiveness: Investing in quality data annotation can reduce the long-term costs associated with model retraining and error correction.
Applications of Data Annotation in Business
The applications of data annotation machine learning span various industries. Here’s an overview of how different sectors utilize data annotation to enhance their operations:
1. Retail and E-commerce
In the retail sector, businesses use machine learning to analyze customer behavior and purchasing patterns. Data annotation plays a vital role by labeling images of products, customer feedback, and transaction data. This information helps in:
- Personalizing marketing strategies
- Optimizing inventory management
- Enhancing customer service through chatbots and automated responses
2. Healthcare
The healthcare industry relies heavily on accurate data for diagnostics and treatment planning. Data annotation is used for:
- Annotating medical imaging data to aid in disease detection
- Labeling patient records for predictive analysis
- Enhancing research through data-driven insights
3. Finance
In finance, data annotation helps in fraud detection and risk assessment. Annotated transaction data is crucial for training models to identify suspicious patterns and anomalies, reducing financial losses.
4. Autonomous Vehicles
The development of self-driving cars relies heavily on data annotation for training algorithms that interpret sensor data. Annotating images and videos helps self-driving systems understand their surroundings, enhancing safety and reliability.
Challenges in Data Annotation
Despite its importance, data annotation presents several challenges. Understanding these challenges can help businesses address them effectively:
- Quality Control: Ensuring high-quality annotations is critical. Poor-quality data can lead to inaccurate models.
- Scalability: As the amount of data grows, maintaining efficient and accurate annotation becomes increasingly challenging.
- Resource Allocation: Data annotation can be resource-intensive, requiring significant time and labor.
Strategies for Effective Data Annotation
To overcome the challenges of data annotation, businesses should consider the following strategies:
1. Employing Skilled Annotators
Hiring experienced annotators who understand the context and requirements of the dataset is vital for achieving high-quality annotations. Training annotators to minimize errors and improve consistency can lead to better outcomes.
2. Utilizing Technology
Leveraging advanced tools and software can streamline the annotation process. Automated annotation tools can assist human annotators, enabling faster processing times and reducing workload.
3. Quality Assurance Processes
Implementing robust quality assurance processes to review annotations can help identify and correct mistakes early, ensuring the dataset's integrity before it's used for training.
4. Continuous Feedback Loop
A feedback loop involving data scientists and annotators can foster continuous improvement. Gathering and acting on feedback can help refine tagging criteria and improve overall performance.
The Future of Data Annotation in Business
As businesses continue to adopt machine learning technologies, the demand for effective data annotation will only increase. The future landscape may include:
- Increased Automation: AI-powered tools may begin to take on more annotation tasks, allowing human annotators to focus on complex labeling that requires contextual understanding.
- Remote Annotating Solutions: The rise of remote work has led to the development of platforms facilitating remote data annotation without sacrificing quality.
- Interdisciplinary Collaboration: Greater collaboration between data scientists, domain experts, and annotators will lead to more effective annotation strategies tailored to specific business needs.
Conclusion
In conclusion, data annotation machine learning is a cornerstone of modern business intelligence and analytics. By investing in quality data annotation practices, companies can enhance the performance of their machine learning models, leading to improved accuracy, better decision-making, and ultimately, enhanced business outcomes. Embracing the potential of data annotation not only allows businesses to harness the power of machine learning but also positions them for success in the ever-evolving digital marketplace.
As organizations like keymakr.com explore opportunities in home services, keys, and locksmiths, leveraging data-driven decision-making enabled by machine learning will become increasingly essential. The businesses that invest in effective data annotation will likely outpace their competition, leading the charge toward innovation and growth in a data-centric world.