Optimizing Business Operations with Data Labeling in Machine Learning
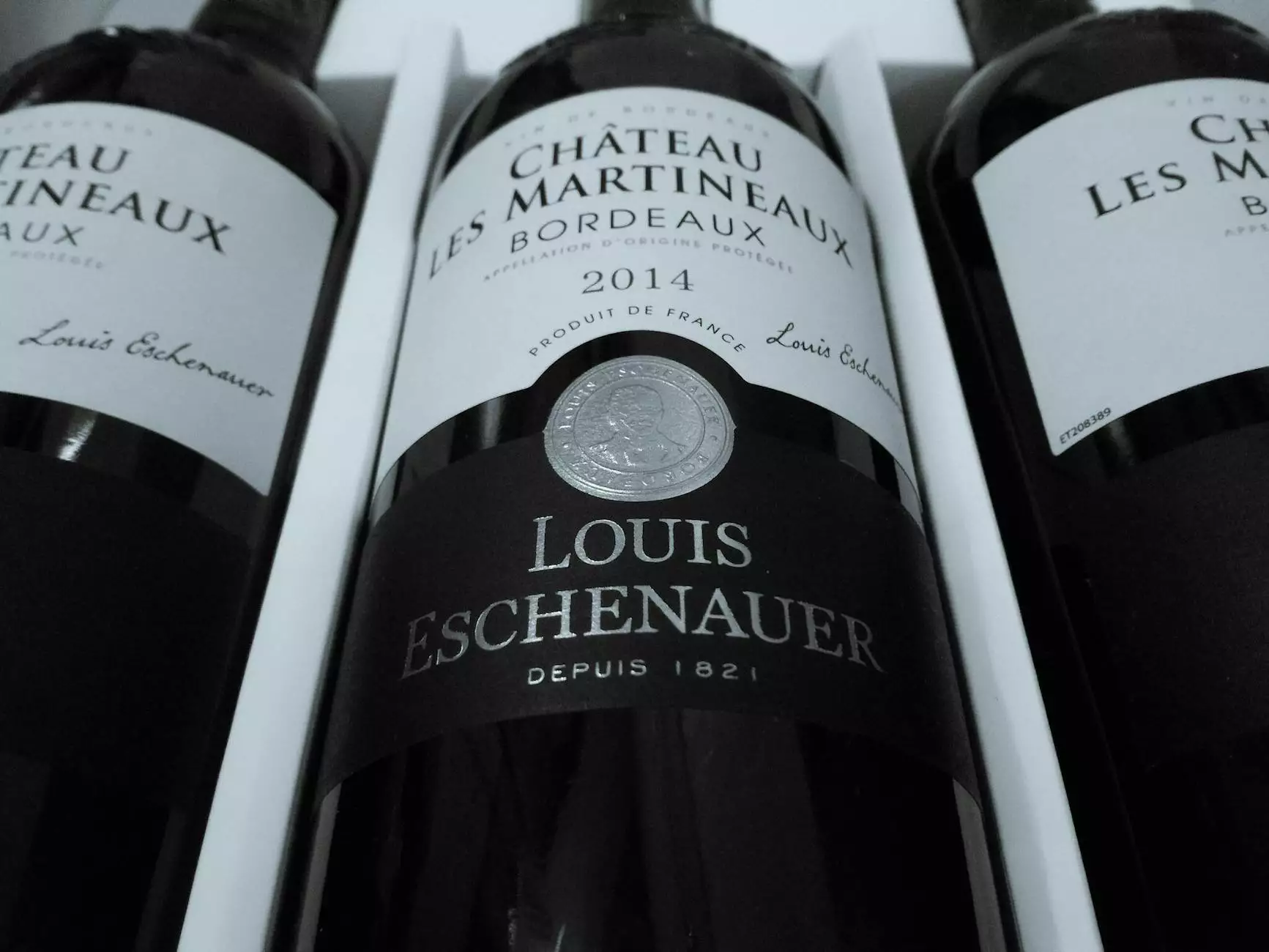
The rise of machine learning has opened a plethora of opportunities for businesses to optimize their operations. However, the effectiveness of machine learning models largely depends on the quality of the data used for training. This is where data labeling comes into play. In this comprehensive article, we will explore the significance of data labeling, particularly in the context of the locksmith industry and home services provided by businesses like Keymakr.
The Importance of Data Labeling in Machine Learning
Data labeling involves the process of annotating data to enable machine learning algorithms to interpret it accurately. This process is crucial for various applications, including image recognition, natural language processing, and more. For businesses, understanding the importance of data labeling can translate to enhanced operational efficiency, improved customer service, and optimal resource allocation.
How Data Labeling Works
Data labeling encompasses several steps to ensure the input data is ready for machine learning models:
- Data Collection: Gathering relevant data from various sources.
- Annotation: Annotating or labeling data accurately. This can involve categorizing images, tagging text, etc.
- Quality Control: Implementing rigorous checks to ensure that the labeled data meets the required quality standards.
- Training the Model: Using the labeled data to train the machine learning model.
- Evaluation: Measuring the model's performance and making necessary adjustments.
Impact of Data Labeling on Business Efficiency
In the context of businesses like Keymakr, which specialize in home services including keys and locksmithing, the implications of effective data labeling are particularly profound:
1. Enhanced Customer Experience
By employing data labeling, locksmiths can better understand customer needs and preferences through data analytics. For instance, analyzing customer feedback can help in designing services that address specific security concerns, leading to higher customer satisfaction.
2. Improved Operational Strategies
Effective data management and labeling can lead to the identification of patterns in service demands. Machine learning models can predict peak times for locksmith services, allowing businesses to allocate resources efficiently, thus reducing wait times for customers.
3. Competitive Advantage
In the highly competitive landscape of home services, companies that utilize data labeling for machine learning are better equipped to make data-driven decisions. This proactive approach not only sets them apart from competitors but also positions them as industry leaders.
Data Labeling Techniques
There are several techniques used in data labeling that businesses should consider, including:
- Manual Labeling: Human annotators label the data, ensuring a high level of accuracy.
- Crowdsourcing: Engaging a large number of people to label data can be cost-effective, but it may introduce variability in quality.
- Automated Labeling: Using algorithms to label data, which can speed up the process, but may require additional verification to ensure accuracy.
Challenges in Data Labeling
Despite its benefits, data labeling comes with its own set of challenges:
- Quality Assurance: Ensuring consistency and accuracy across labeled data can be difficult, especially with large datasets.
- Time-Intensive: The manual labeling process can be time-consuming, leading to delays in the machine learning model's deployment.
- Cost Implications: Depending on the volume and complexity of data, the resources required for effective labeling can be significant.
Best Practices for Effective Data Labeling
To overcome the challenges and harness the full potential of data labeling, consider the following best practices:
- Establish Clear Guidelines: Creating comprehensive labeling guidelines can help maintain consistency across various datasets.
- Invest in Quality Control: Implementing a robust quality control process ensures that the labeled data meets the necessary standards.
- Leverage Technology: Utilize data labeling tools and software to streamline the process and reduce human error.
- Regular Training: Training annotators consistently on the evolving needs of the business can help enhance the quality of the labeling process.
Conclusion
In conclusion, data labeling in machine learning is an indispensable aspect of the modern business landscape, particularly for industries that demand precision and efficiency, such as home services and locksmithing. By understanding and implementing effective data labeling strategies, businesses can significantly enhance their operations, improve customer satisfaction, and achieve a distinct competitive edge. In an era where data reigns supreme, investing in high-quality data labeling processes will undoubtedly pave the way for future success.
For businesses like Keymakr, embracing such methodologies not only prepares them for the challenges of today but also equips them for the innovations of tomorrow. As machine learning continues to evolve, those who prioritize data labeling will find themselves at the forefront of their industries.
data labeling machine learning