The Ultimate Guide to Semantic Segmentation Labeling Tools
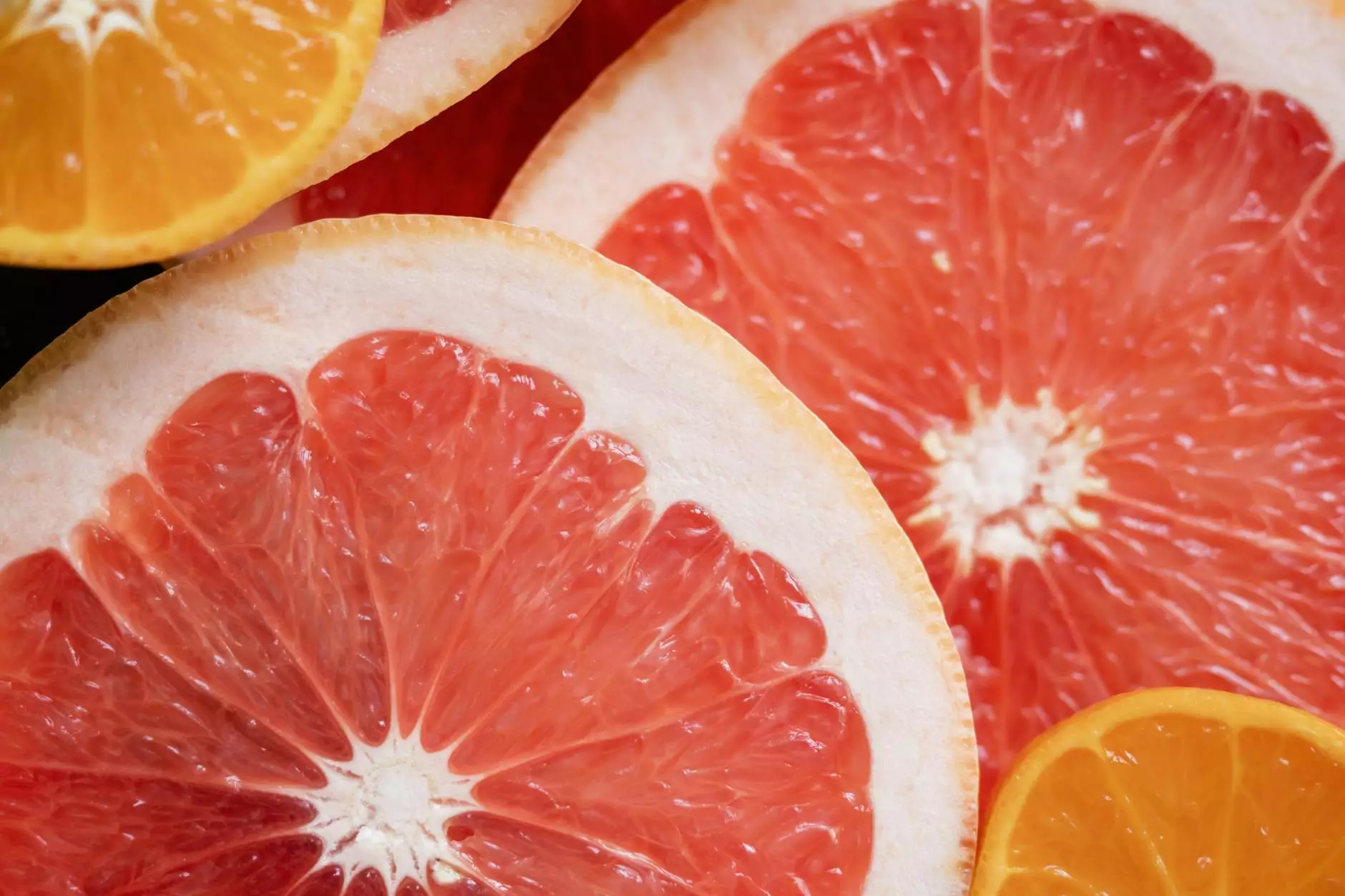
In today's rapidly evolving world of artificial intelligence (AI) and machine learning (ML), data is the driving force powering innovations. Semantic segmentation labeling tools play a crucial role in ensuring that AI models can understand and interpret visual information with precision and accuracy. In this comprehensive guide, we'll explore the features, benefits, and applications of these tools, while highlighting how KeyLabs.ai stands out as a premier data annotation platform.
Understanding Semantic Segmentation
Semantic segmentation is a process in computer vision that involves categorizing each pixel in an image into a specific class. Unlike traditional image classification, which assigns an entire image to a category, semantic segmentation provides a much finer granularity by allowing models to distinguish between different objects in an image. This makes it essential for tasks such as autonomous driving, medical imaging, and robotic vision.
The Importance of Data Annotation in Machine Learning
Data annotation is the backbone of machine learning, especially for supervised learning tasks where a model learns from labeled data. High-quality labeled datasets enable ML models to make accurate predictions. Here’s why data annotation, particularly through semantic segmentation labeling tools, is vital:
- Enhanced Model Accuracy: Properly annotated data helps models achieve higher accuracy in object detection and recognition tasks.
- Improved Training Efficiency: Comprehensive datasets reduce the need for extensive retraining and tuning.
- Real-World Applications: From healthcare to automotive, accurate segmentation is crucial for practical deployments.
Key Features of a High-Quality Semantic Segmentation Labeling Tool
When selecting a semantic segmentation labeling tool, it is essential to consider its features. A robust tool should include:
1. User-Friendly Interface
The interface should be intuitive and accessible, allowing users to annotate images seamlessly. A well-designed UI minimizes the learning curve and accelerates the annotation process.
2. Multi-Format Support
A quality tool should support various image formats and data types, enabling extensive versatility for different projects.
3. Collaborative Features
Collaboration is key in data annotation, especially for large teams. Features that allow multiple users to contribute to labeling tasks in real-time can significantly speed up project timelines.
4. Automated Annotation Capabilities
While manual annotation is often necessary, tools that incorporate AI-assisted features can help automate parts of the process, reducing the time and effort involved.
5. Quality Assurance Mechanisms
Quality control is crucial when annotating data. High-end tools provide mechanisms to verify and validate annotations to ensure data integrity.
KeyLabs.ai: A Leader in Data Annotation Platforms
KeyLabs.ai excels in providing a comprehensive data annotation tool that implements all the essential features discussed above. Here’s why it stands out:
Integrative AI Technology
KeyLabs.ai leverages cutting-edge AI technology to enhance the annotation process. Its semantic segmentation labeling tool utilizes machine learning to facilitate quicker and more accurate labels. Users can upload image datasets and benefit from advanced ML algorithms that assist in pixel-wise labeling.
Robust Collaboration Tools
With KeyLabs.ai, teams can work together seamlessly, whether they are in the same location or across the globe. The platform allows multiple users to annotate simultaneously, ensuring rapid progress on projects without compromising on quality.
Streamlined Workflow and Project Management
The platform features integrated project management tools that help users organize their workflows efficiently. Users can track project milestones, manage deadlines, and allocate tasks to team members effortlessly.
Unmatched Support and Training
KeyLabs.ai provides exceptional customer support and resources to help users maximize their experience with the semantic segmentation labeling tool. Comprehensive tutorials, documentation, and direct support channels ensure that users can quickly overcome any challenges they face.
Applications of Semantic Segmentation Labeling Tools
The versatility of semantic segmentation labeling tools makes them applicable across numerous fields. Here are some notable applications:
1. Autonomous Vehicles
In self-driving car technology, understanding the road environment is critical. Semantic segmentation helps identify road signs, pedestrians, vehicles, and lane markings, enhancing the safety and reliability of automated transportation.
2. Healthcare and Medical Imaging
In medical imaging, semantic segmentation is used to delineate structures in images, such as tumors in MRI scans. Accurate segmentation aids in diagnosis, treatment planning, and monitoring of diseases.
3. Agricultural Monitoring
Farmers utilize visual data from drones and satellites to assess crop health. By employing semantic segmentation tools, they can identify weed infestations, crop species, and health status, enabling precise interventions.
4. Retail and Inventory Management
In retail, semantic segmentation can be applied to analyze customer movement within stores. Insights gained can inform layout optimization and marketing strategies.
Challenges in Semantic Segmentation and How to Overcome Them
While semantic segmentation is powerful, there are notable challenges:
1. Data Quality and Diversity
One of the primary challenges is ensuring a diverse and high-quality dataset. Collections must be representative of the scenarios the trained models will operate in.
2. Time-Consuming Annotation Process
Manual annotation can be painstaking. Utilizing automated features and efficient workflows, like those offered by KeyLabs.ai, can significantly reduce the time required.
3. Continuous Model Training
Models require ongoing training with new data to maintain accuracy. Establishing a systematic data collection and annotation routine is crucial to address this issue.
Conclusion
Semantic segmentation labeling tools are invaluable assets in the world of data annotation, powering advancements in technology and industry. By leveraging tools like KeyLabs.ai, businesses can harness the power of quality annotated data to improve their machine learning projects significantly. As we continue to move towards a more AI-driven future, the significance of these tools will only grow, solidifying their role as essential components of effective data management practices.
For organizations looking to elevate their data annotation processes, investing in a high-quality semantic segmentation labeling tool is an investment in the future. Discover the potential of efficient and accurate data annotation with KeyLabs.ai and position your projects for success in the competitive landscape of AI and machine learning.